Beyond Dashboards – The Era of Digital Twins in Power Systems
Digital twins have emerged as transformative tools across various domains, particularly in energy systems. This article explores the evolution of digital twins from virtual replicas to autonomous decision-support tools, focusing on their applications in power system analytics, prediction, optimization, and decision support, enabled by dynamic models and real-time data.
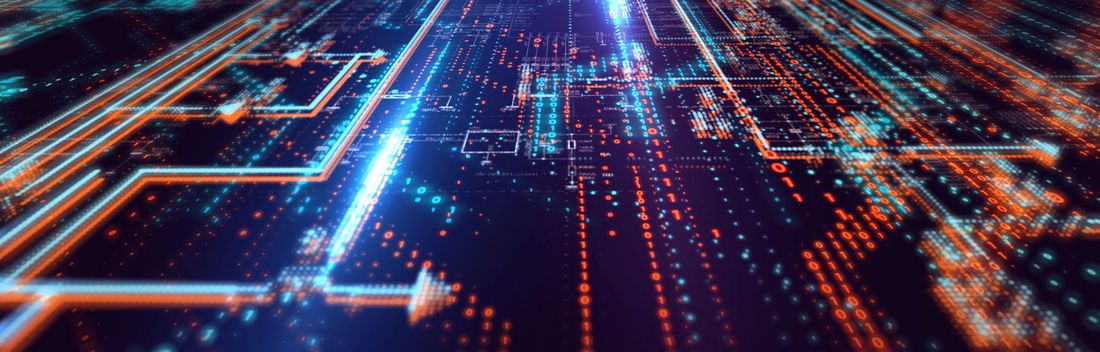

CEO, Minaatral Power Systems, India
Introduction
Digital twins are sophisticated virtual replicas of physical assets, systems, or processes, typically consisting of the following three components.
- A dynamic model of the asset or the system.
- An evolving set of data that continuously updates the model
- A means for deriving actionable insights, operational strategies, or optimization methods.
Digital twins hold different meanings for different stakeholders. Equipment manufacturers might leverage them for lifecycle analysis and next-generation asset design, while utilities and system operators use them for grid integration and long-term asset management. Maintenance teams could focus on predictive maintenance and failure analysis. This wide array of applications highlights the importance of clearly defining objectives and use cases before implementation.
This article delves into how digital twins can evolve beyond diagnostic tools, advancing into autonomous systems that integrate real-time data with expert knowledge to deliver contextual situational awareness and informed decision-making.
Dynamic Hybrid Modelling
The implementation of the digital twin starts with the model definition of the asset that captures its features and parameters. It is important to select the right model based on the application. The selected model also needs to have the following characteristics to ensure optimal performance and reliability.
- Accurate: The model should closely replicate the behavior of the physical asset. In addition to defining the model error and accuracy, statistical methods such as uncertainty quantification can improve the trustworthiness of the model.
- Generalized: The model should be flexible enough to represent various operational conditions and scenarios without requiring significant modifications. This helps the digital twin to maintain relevance across different use cases with the power system.
- Efficient: The model should reduce the computational burden to ensure we can derive near-real-time insights and that the twin is in sync with the physical asset. High computational efficiency is crucial in enabling faster decision-making and responsive control actions.
- Dynamic: The model should be able to learn and evolve based on real-time data and new events. This plays a pivotal role in the continuous calibration process of the digital twin, which allows the digital twin to enhance its predictions over time.
Digital twin models are generally classified either as physics-based or data driven, with the former dealing with the physics of the equipment and the phenomena it experiences. Data driven models deal with data mining, manipulation and fusion, to derive various insights about the assets. Hybrid models, such as Physics Informed Neural Networks or PINNs combine the foundations of physics with the computational efficiency of data driven models by integrating the laws of physics directly into the neural networks. An added benefit is the independence from huge amounts of training data, which helps in training the PINN with sparsely available or even simulated data. Integrating this with real-time information helps in providing near real-time insights and in continual refinement of the model, making it dynamic and adaptive to unseen situations. While traditional PINNs use the known physics to make inferences, inverse PINNs (IPINNs) leverage the observed data to infer the underlying physics and its parameters, by solving the inverse problem. The knowledge from these trained models can also be used in the digital twins of newer assets through transfer learning, ensuring that the updated information is always utilized.
Let us take a case of a digital twin model for estimating the dynamic overloading of transformers, which balances increasing transformer utilization efficiency with maintaining high system reliability. PINNs can model the thermal behavior of the transformer by incorporating the physics of heat dissipation in the transformer oil to estimate the hotspot temperature from the real time inputs such as loading and ambient temperature. Top oil temperature measurement can be used for calibration of the model by comparing the estimated and measured value.
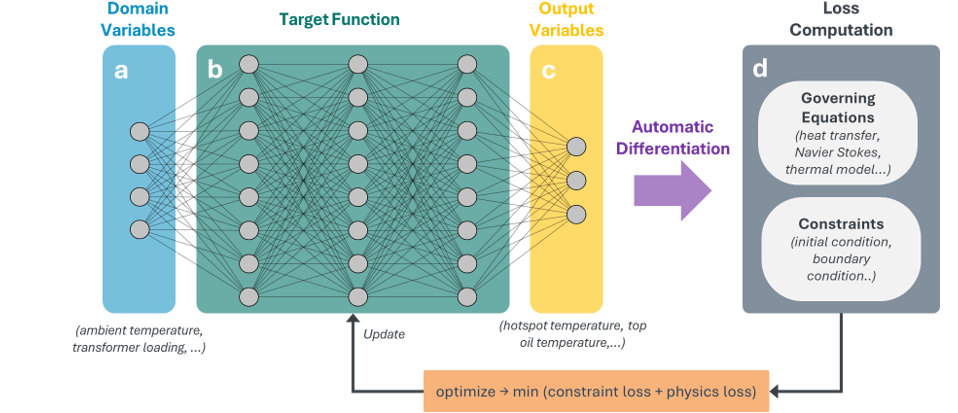
Figure 1 - PINN Architecture
“What-if” Scenario Management
The behavior of the physical asset under different 'what-if?' scenarios should be integrated into the analytics. The ability of the digital twin to perform scenario analysis helps it move from predictive to prescriptive decision making. Currently, running manual scenarios by varying the parameters and conditions is a time-consuming process, which is subjective to expert knowledge and bias, drastically limiting insights. To provide truly autonomous decision making, the digital twin should be capable of managing multiple scenarios and combining them with online real-time learnings. A dynamic and hybrid model of the asset plays a major role in providing optimal decision support to the users.
To illustrate the potential of advanced scenario management in the case of transformer overloading, digital twins can be used to generate multiple scenarios of loading categories, weather conditions and grid configurations. The thermal behavior and remaining useful life (RUL) of the transformer is analyzed under multiple scenarios, providing the user with risk assessment and uncertainty quantification of accelerated aging and thermal limitations of the transformer. This can lead to recommendations for improved operational and maintenance strategies that maximize asset utilization while maintaining acceptable risk levels. Operators can use also the digital twin to explore hypothetical “what-if?” scenarios, such as the impact of upgrading the cooling system or sizing considerations.
Human-in-loop AI driven Solutions
For critical infrastructure such as power systems, digital twin technologies shall apply the concept of human-in-loop solutions. This fosters trust in how users and stakeholders engage with the digital twin.. While digital twins provide recommendations by processing huge amounts of information, human judgment remains essential in critical decision-making processes. The output of the digital twin shall be accompanied by decision support rules in the form of risk assessments, uncertainty quantification and statistical analysis. The feedback from the user can also be used to refine the digital twin model providing better support over time.
While autonomous decision-making is the ultimate objective, achieving it requires a high level of maturity in both systems and operators.
The Next Generation of Digital Twins
The evolution of digital twins is ushering in a new era where we move beyond static dashboards and manual analysis toward interactive, AI-driven tools. Much like how conversational AI systems allow users to engage with vast datasets through natural language interfaces, the next generation of digital twins will transform how we interact with power systems. Rather than just presenting data, these systems will act as intelligent agents, offering real-time insights, autonomously exploring 'what-if' scenarios, and providing explanations, predictions, and optimized strategies, based on user queries, that mimics human conversation.
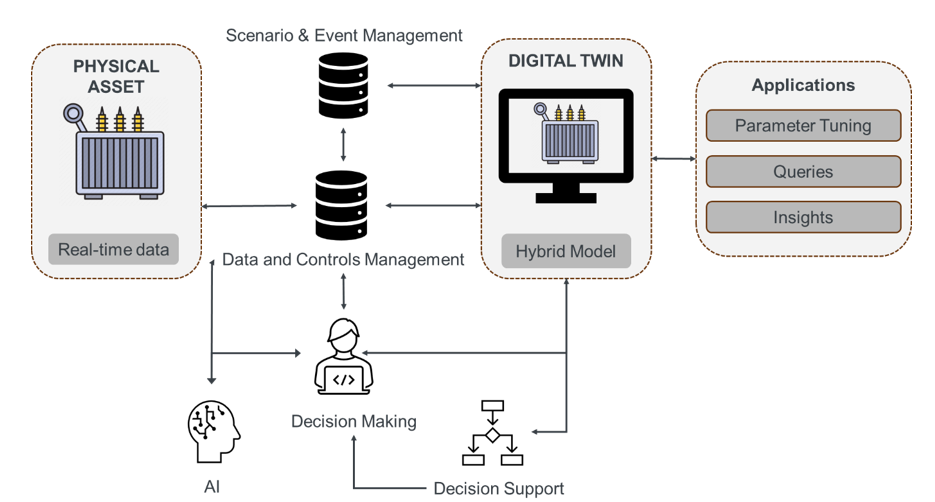
Figure 2 - The Next Generation of Digital Twins
Conclusion
As these digital twins become more dynamic and capable, they will empower users to make faster, data-driven decisions with greater confidence. The future lies in collaborative intelligence, where digital twins evolve into integral components of power system management, providing deeper situational awareness and smarter, more efficient solutions.
Banner & thumbnail credit: spainter_vfx on iStock